Operationalizing data quality means making quality improvements accessible and actionable. Using metadata, you can establish quality standards, understand where your current data falls short, and map the improvement activities that will take it to your desired state.
For some organizations, data is scattered across different systems and departments. This often leads to fragmentation, inconsistency, and inaccuracy — making it difficult to establish centralized data quality processes and controls.
In our recent webinar, data management expert Olga Maydanchik joined Shinji Kim, founder and CEO of Select Star, to delve into strategies for embedding data quality into the day-to-day activities of your organization.
A Foundation of Data Quality
Data quality is inextricably linked to data literacy across all levels of an organization.
Olga said data literacy is required regardless of vendor and industry. The level of proficiency needed within a team correlates directly to individual roles, so organizations must institute a comprehensive program to evaluate and enhance individual competency levels.
Traditional Metadata vs. Active Metadata Management
Traditional metadata management relies on manual updates and interventions by data stewards. Active metadata management employs automated processes and real-time updates to ensure instant synchronization and drive proactive data management.
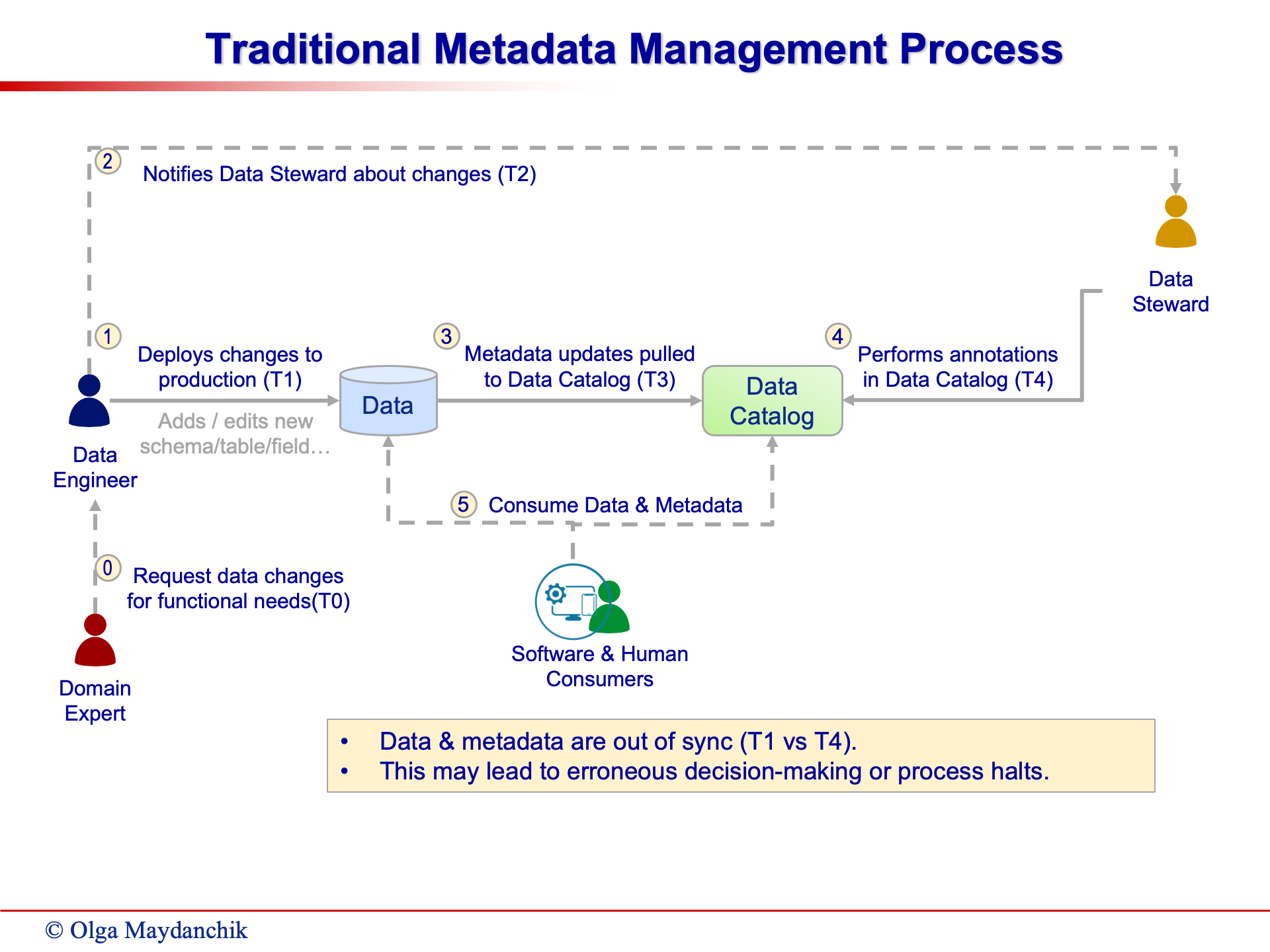
Traditional Metadata Management
Traditional metadata management involves users manually annotating and disseminating metadata.
This manual intervention introduces delays and increases the risk of data being out of sync with the metadata catalog.
Active Metadata Management
With active metadata management, gone are the days of manual interventions and delayed updates. With automated processes and real-time APIs, every change to metadata triggers instant updates, ensuring that data remains in sync across the ecosystem.
Olga said active metadata represents a paradigm shift in how we leverage metadata within data ecosystems. It's not just about cataloging data — it's about using metadata to drive actionable insights and real-time decision-making.
"Active metadata is a way of using metadata to power down other systems," she explained.
Shinji emphasized that active metadata involves more than just data itself. It involves processes, functions, or programs that react based on metadata, enabling a proactive approach to data management and governance.
In essence, active metadata becomes a vital part of the entire system.
Consider the example of policy-based access control. With active metadata, whenever a new field is added to a table or a new table is introduced, access control policies seamlessly adapt to accommodate these changes.
Without active metadata, access management would struggle to determine how to treat new data elements, potentially disrupting the entire system.
To get the most out of active metadata, your teams need to learn how to manage it.
How Active Metadata Impacts Data Quality
Active metadata impacts data quality by streamlining data processes, automating tasks such as classification and tagging, reducing manual intervention, and minimizing the risk of errors.
It directly translates into significant ROI, as it not only enhances operational efficiency but also reduces costs associated with errors — maximizing overall business value.
7 Active Metadata Use Cases
Active metadata management drives business impact across organizational operations:
- Machine Learning Data Classification
- Active Metadata in DQ Error Resolution
- Data Governance
- Root Cause Analysis
- Data Observability
- Profiling
- ETL or schema changes
1. Machine Learning Data Classification
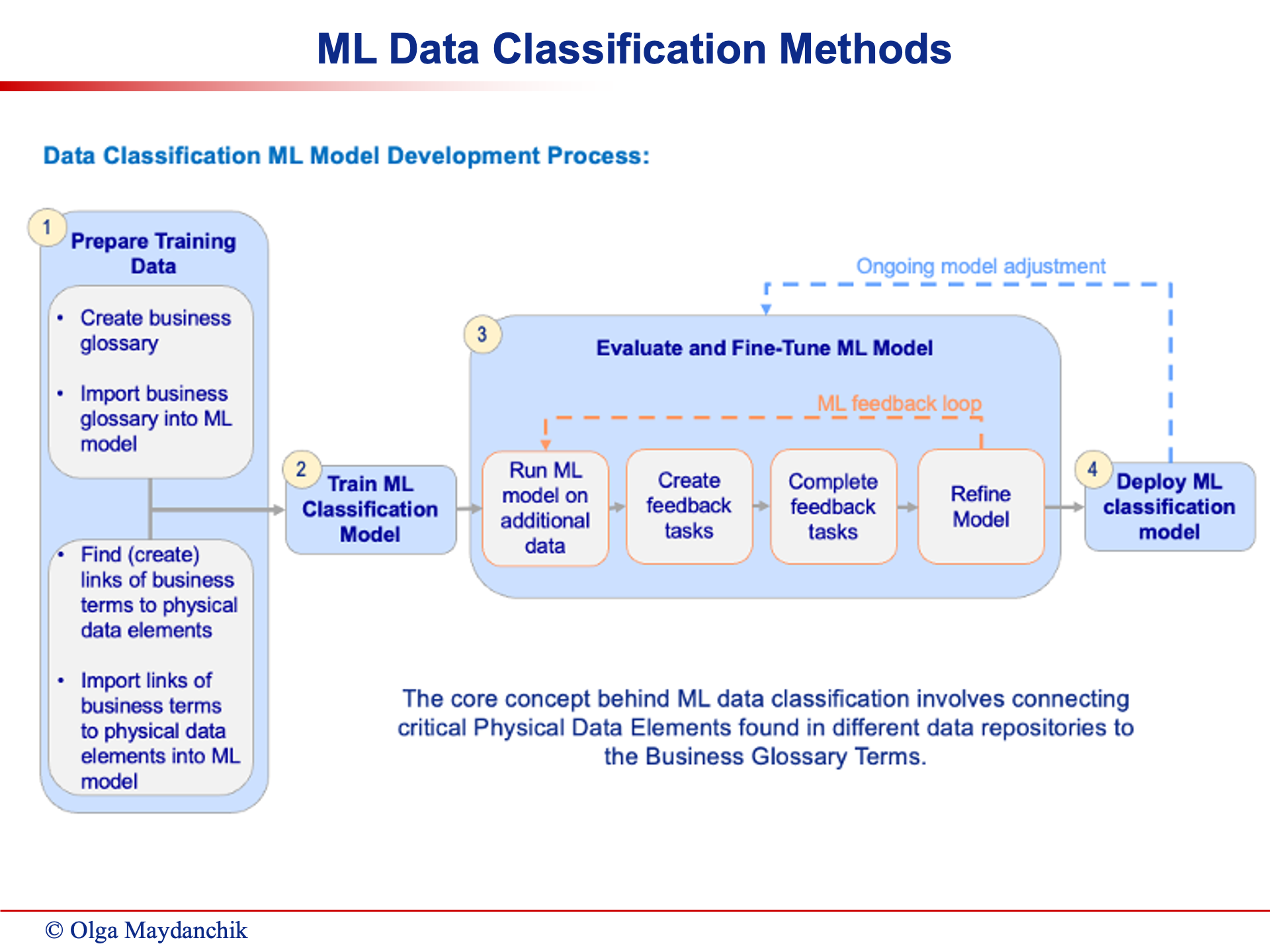
One key advantage of active metadata is its ability to facilitate a feedback loop, making it an excellent approach for machine learning.
Through continuous learning and refinement, the machine-driven program can enhance its classification capabilities over time, resulting in more precise and reliable data categorization.
Active metadata management ensures seamless execution of data classification processes. Once initiated, the program can execute automatically every time new data is introduced, minimizing manual intervention and streamlining data management workflows.
2. Active Metadata in DQ Error Resolution
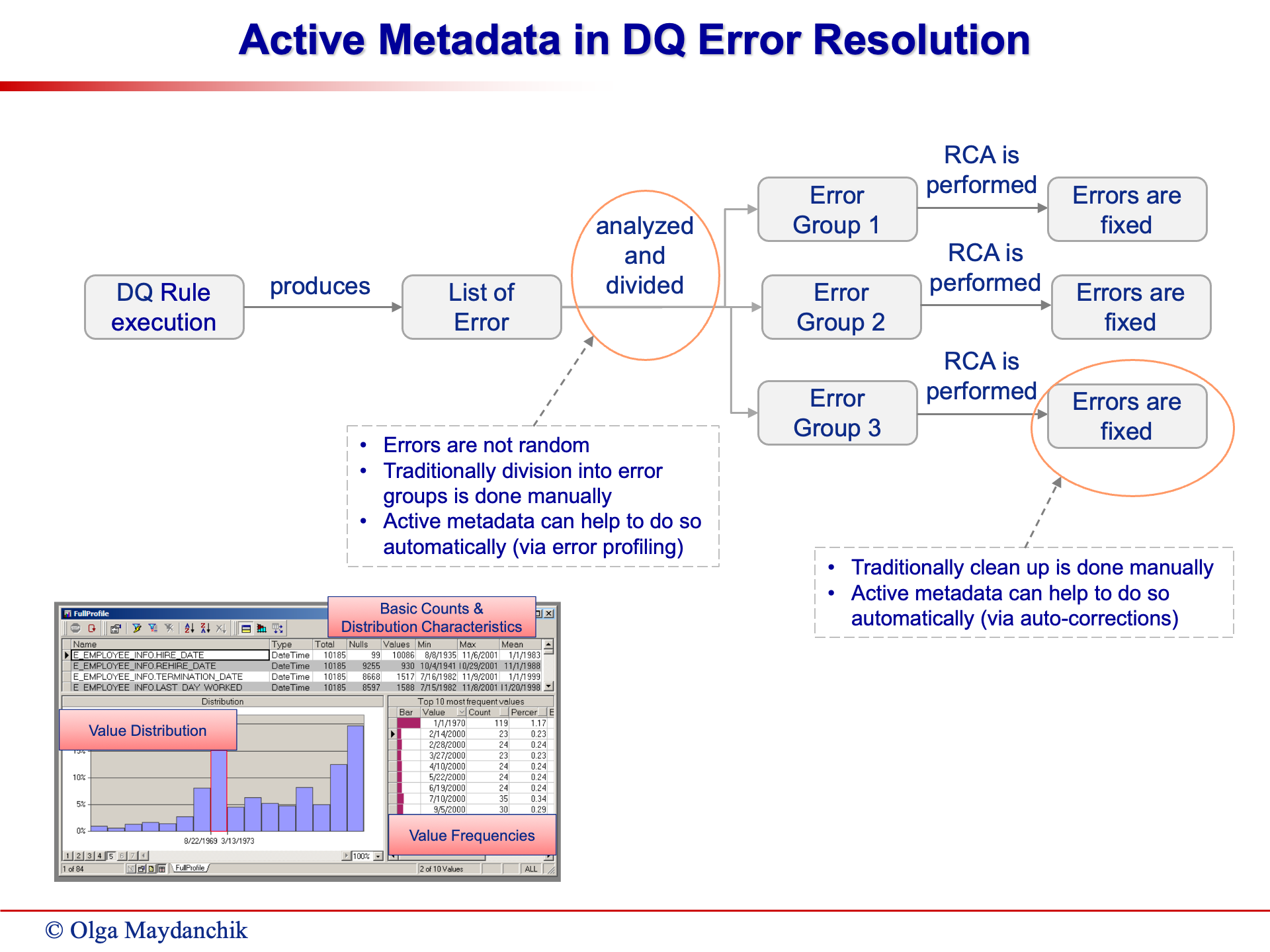
Active metadata usage extends beyond error detection to error resolution. This operation involves the analysis and categorization of errors into groups, identifying underlying issues, and ideally automating the resolution process.
3. Data Governance
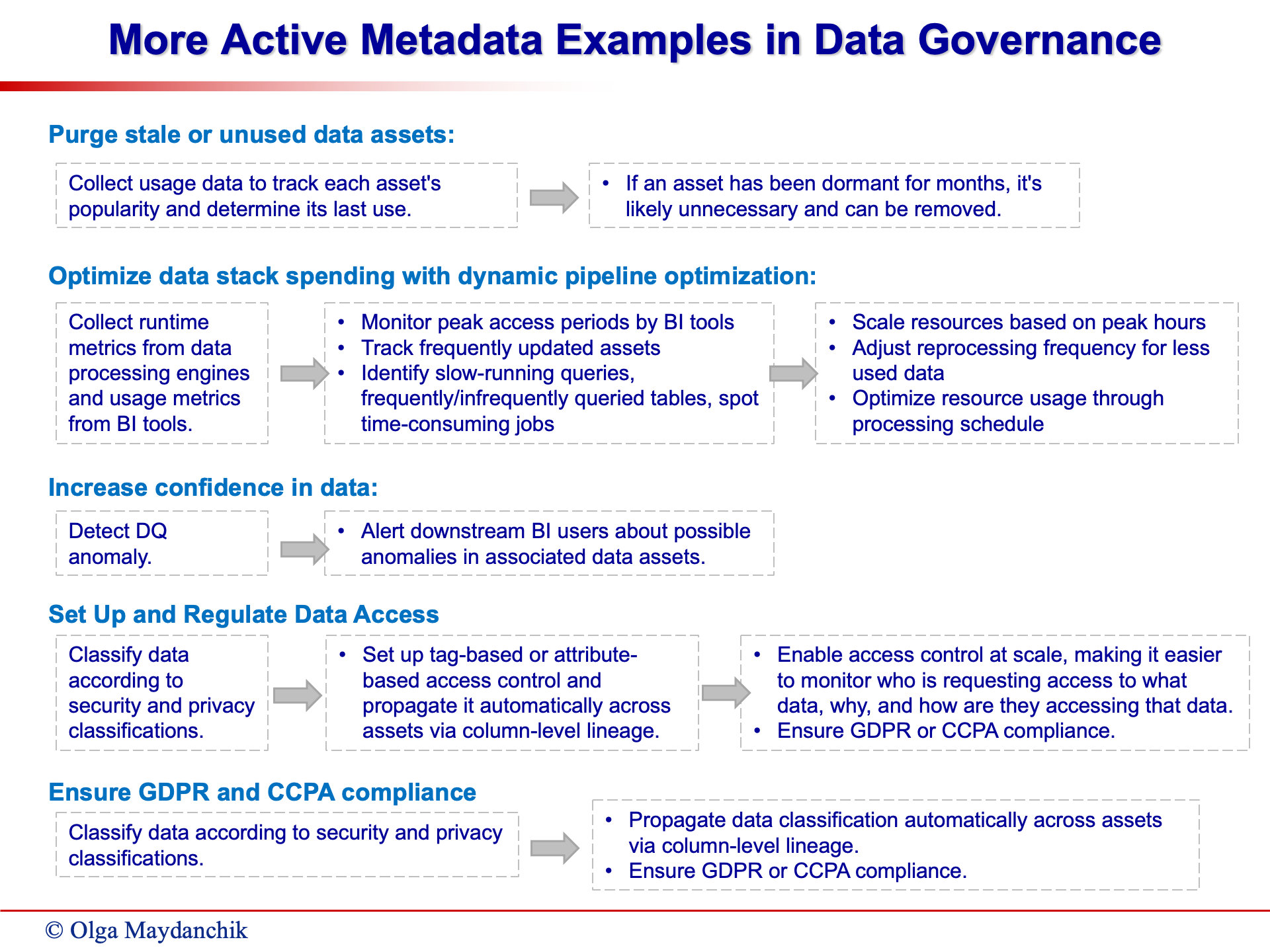
Olga highlighted how active metadata facilitates pipeline optimization by collecting runtime metrics, monitoring access patterns, tracking asset updates, identifying performance bottlenecks such as slow-running queries, and pinpointing time-consuming jobs.
Active metadata enables organizations to optimize data utilization, streamline storage, and enhance operational efficiency. Your teams can automate the deletion of unused data, reducing storage costs and minimizing data clutter.
4. Root Cause Analysis
Root cause analysis often demands significant engineering time and resources, especially when issues impact critical systems or revenue streams, such as models that serve external customers.
Active metadata expedites the resolution process. Active metadata furnishes engineers with comprehensive insights, enabling them to identify and address root causes in minutes rather than hours.
5. Data Observability - Reporting and Error Resolution
Active metadata enables organizations to calculate extensive statistics about their metrics using data observability reports.
"Data quality is expensive and very time-consuming,” Olga said. “Active metadata helps you to find those very little pieces of how you could do it."
Shinji said a common example is when modern observability players conduct anomaly detection to see whether there is an unusual error.
6. Profiling
Profiling is another useful case for metadata, particularly for datasets originating from user input sources, Shinji said.
Consider scenarios like quality checks on documents, where it’s imperative to preprocess user-generated data before it enters a more structured environment.
Active metadata helps you conduct comprehensive profiling to identify potential anomalies and ensure quality before data is integrated into critical systems or processes.
7. ETL or Schema Changes
As data becomes increasingly democratized, analysts and engineers may create different models on top of one another — necessitating changes either at the product level or within the source data. These changes can have far-reaching implications, potentially impacting downstream reporting tables and other models.
Shinji emphasized the importance of monitoring schema changes throughout the data pipeline as a means of addressing these changes efficiently.
Active metadata facilitates real-time tracking of schema changes, providing valuable insights into the evolution of data structures and facilitating proactive management of ETL processes.
Operationalizing Data Quality
The journey toward operationalizing data quality requires a combination of technological innovation and data-literate culture.
Olga noted continuous learning is essential. She recommended exploring resources from thought leaders at companies like Eckerson Group and leveraging platforms like eLearningCurve for in-depth classes taught by industry experts.
She also dedicates time to reading insightful books that enrich her understanding of data management and quality practices.
By embracing a commitment to ongoing education and partnering with experts, organizations can drive success in the data-driven era.
To discover how your organization can benefit from improving data quality practices, book a demo.